Traditional character recognition’s shortcomings can prevent your organization from achieving peak operational efficiency. Without the ability to reliably deliver accurate data, their promises of automation are feigned, ultimately pushing errors downstream that need to be corrected by humans. Hyperscience takes a fundamentally different approach that helps you achieve the highest levels of accuracy and automation on the market.
“We chose Hyperscience because they are freaking amazing and can do magical Machine Learning [data] extraction.”
Matt McGinley, CIO, Precision Medical Products
Accuracy makes all the difference when it comes to automatically classifying documents and extracting the critical information they contain for downstream usage and decision-making. A wrong digit extracted from a new mortgage application at the start of the document’s journey can create time-consuming, costly delays downstream. Ultimately, the error can result in an individual not being approved for the mortgage loan.
That is why Hyperscience was built to prioritize accuracy out of the gate.
But what does that mean and how do we ensure performance in practice?
The Technology Behind the Magic
“I’ve been in technology 20 years and this is the first time technology is magic.”
Global Insurance and Asset Management Company
Hyperscience defines automation as the amount of work the machine does without human intervention. The best way to avoid unnecessary human intervention – and manual review oversight due to errors making their way downstream – is leading with accuracy.
Focusing on accuracy, and developing a robust, proprietary data extraction engine first and foremost, is core to our approach. It’s the critical first step towards unlocking meaningful, longtail intelligent automation and efficiency gains.
Hyperscience uses Deep Learning techniques to build and train our own neural networks on a vast number of real world samples. This allows our neural networks to overcome the inherent nuances and complexities of real-world document processing, like the ones OCR struggles with, out-of-the-box. The more data a model is exposed to, the more the network can learn and improve its accuracy, driving fewer error rates and higher automation
At the same time, our built-in Quality Assurance mechanism runs in the background to drive performance improvements.
Our built-in Quality Assurance mechanism uses consensus to establish what we call “Ground Truth”. When two parties agree, a consensus is reached as pictured below. The data agreed upon is used to train the solution about its confidence. Confidence is the machine’s ability to know when it is right and when it is wrong; ultimately resulting in better decision-making when it comes to flagging a field for human review and resolution.

Hyperscience customers set their desired accuracy target based on internal Service-Level Agreements or other compliance criteria. Once set, Hyperscience extracts data accordingly and automates as much of the work as possible. In general, a higher accuracy target will lead to lower automation. That is because the platform will only automate when it is confident it can reach the target accuracy.
Our customers can expect over 80% automation at over 98% accuracy on day one with additional improvements over time.
Legacy data capture tech, or OCR products, aren’t as accurate or robust in their capabilities at the start. They also lack the intelligent ML and Deep Learning AI tech to improve over time.
Why 100% Automation is Dangerous
If a legacy vendor promises 100% automation, you should be skeptical. They’re most likely pushing through errors that cause more headaches, rework, and cost downstream. If you get near 100% automation with 55% accuracy, what good is that automation?
What’s more, this erodes downstream confidence in your users, leading them to double-check the machine’s work and or redo the work without even seeing what the machine’s output is. In addition, without reliable data, you can’t automate adjacent steps in a process to drive decision-making or outcomes.
In some cases, enterprises we work with that have historically relied on outdated, legacy tech built cumbersome manual workarounds to account for the inherent inaccuracies and document processing limitations. So much for automation!
Fortunately, where OCR falls short, the Hyperscience Intelligent Document Processing [IDP] solution excels.
How Hyperscience Transforms Classification and Data Extraction
“You all don’t optimize data capture – you transform it.”
Canadian Multinational Financial Services Company
Good data extraction begins with correctly classifying documents.
Businesses run on unstructured document data flowing into the organization from outside and within. Think of all the various application or invoice formats you’ve seen. Without a global standard for most document types, it can be hard to know how to read and find the critical information you want to extract off the page. This initial classification step can be slow and resource-intensive with the wrong tool in place.
Users need to train an OCR system, for example, on each template type (read: each document input variation). Without doing so, the document won’t be correctly classified. The solution also won’t know what field to look for and where to find it.
In contrast, Hyperscience does not use zonal or template-based extraction. You can train a single model to handle all variations of common document types, or field ID model, with as few as 400 sample documents.
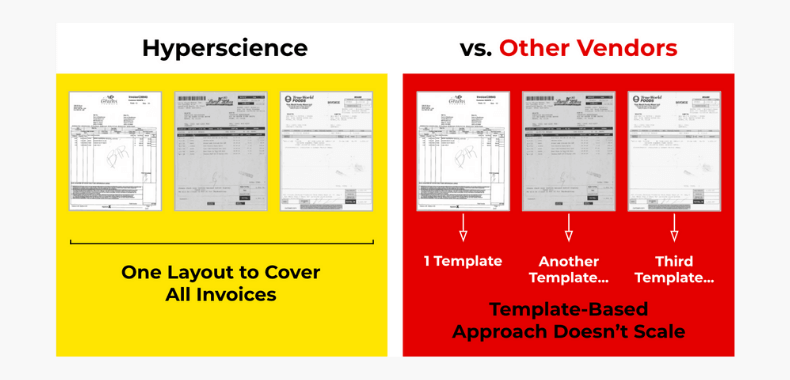
Hyperscience processes pages at the field versus character level, and we measure data accuracy and automation accordingly. When it comes to using this data to drive business outcomes, it’s the overall field result that matters (i.e. is this individual’s name Jonn or John).
Additionally, Hyperscience is intelligent enough to drop out the details of the template, such as “First Name”, and only return the data of interest (“Frank”). Rules-based tech does not. Data keyers have to manually delete any unwanted data extracted before it can be used for further processing. Similarly, we trained separate models to handle each data type. This avoids ambiguity and edge cases- like the system thinking something is a “5” vs. an “S”.
One of the key differentiators between traditional character recognition technology and Intelligent Document Processing is the ability to reliably read handwritten text. Humans have different handwriting styles that make it near impossible to create a single rules-based algorithm to determine if something is a “1” vs. 7” or an “0” vs. “O.”
“The high pull-through rate, the quality that we were getting on specifically handwritten documents, really is what led us down the path of exploring Hyperscience.”
Sean Van Moorleghem, Managing Director, TD Ameritrade
Solving for handwriting requires a leading solution that is able to read and comprehend documents with cursive, scribbles, and messy handwriting with ease. “You have the best handwriting interpretation examples we’ve seen.” – Head of Automation Solutions, American Bank Holding Company.
We also use a single extraction model for handwritten and machine-printed text. Legacy solutions have separate models that require an operator to go into the platform and select which model to use, further limiting scalability and overall automation.
What’s more, humans don’t always write inside the box. Think about the last time you filled out a handwritten form. Did your email or information fit perfectly in the box, or did it differ between lowercase and upper case and get sloppier as the page went on? We’ve trained our solution to read outside the box, enabling stronger data extraction performance.
Our models know that a Social Security Number should be nine digits, for example. So, if the machine only sees eight digits in the field box, it will gradually expand the crop until the missing ninth digit is found.