ML, AI, RPA, IDP, ICR, OCR. . . the world of enterprise technology is changing quickly. It can be hard to keep up and understand the meaning of and difference between each of these acronyms. What exactly are the benefits of IDP vs. OCR? Whether you’re a director of operations upgrading document processing workflows or part of your organization’s digital transformation team, we’re here to be a resource as you make sense of the evolving automation landscape.
If you spend too much time in the alphabet soup of automation technologies, one acronym can start to sound a lot like another. One question we frequently hear from organizations is how our Intelligent Document Processing [IDP] offering differs from traditional optical character recognition [OCR] solutions. Others want to know if IDP is just the newest iteration of OCR. Because most IDP solutions incorporate some form of OCR, it’s easy to see why the lines blur.
So what is IDP? And what is OCR?
- Optical Character Recognition refers to software that can turn a scanned image into text, typically by transcribing it character by character (hence the name). OCR has been around for decades, and as the technology has evolved to include extraction of text from hundreds of languages, so has its name. An offshoot of OCR is Intelligent Character Recognition (ICR). ICR works like OCR, except with the purpose of capturing handwritten characters, one-by-one. ICR relies on a “constrained handprint” that separates handwritten characters into individual boxes. Most forms are not designed for ICR, making automation difficult; more often than not, ICR stumbles when faced with normal handwriting or cursive, requiring manual data entry.
- Everest Research Group defines Intelligent Document Processing as “any software product or solution that captures data from documents (e.g., email text, PDF, and scanned documents), categorizes, and extracts relevant data for further processing using AI technologies.” Leading IDP solutions have tech baked in to increase the quality of the scanned documents (like noise reduction, for example), capture the data, and classify the data and document. These solutions can typically be integrated with internal applications, systems, and other automation platforms, and find a wide variety of use cases across different business functions and verticals, such as claims processing, client onboarding, and record management compliance.
To better understand the difference between the two technologies, we outlined the journey of some hypothetical documents traveling through an organization looks like as IDP vs. OCR.
IDP vs. OCR
OCR simply transcribes a document, leaving you with a text representation of the image, but does not provide the content needed for downstream processes. Also, different document types present challenges for processing through OCR. An IDP solution like Hyperscience is more than just OCR, it is built to identify the business data from a document, and built to deal the real-world challenges different documents may present, like the following common examples:

PDF Invoice, which is machine-generated with printed text and is from an invoice template that is frequently seen at the company.
- For a PDF invoice, most OCR tools will either use the text layer and not perform OCR at all; use the text layer to assist OCR; replace the text layer if it was not electronically-generated (i.e. – they don’t trust whatever OCR software made the PDF searchable).
- Despite the variability of invoices, Hyperscience utilizes multiple tools to capture the data from the document, classify it appropriately, and extract and structure the data, which can be sent downstream for further processing.

Scanned bank account application, which was filled out by hand and was slightly skewed when it arrived at the bank for processing.
- Despite the fact that nearly half of documents are still handwritten, the OCR/ICR engines cannot handle the variability and messy handwritten text, meaning employees have to review and then enter all the data by hand.
- Hyperscience improves image quality of each page automatically and then classifies documents based on user-defined taxonomies. We utilize our own extraction engine, built on modern concepts of computer vision and deep learning models, to transcribe data from the document and structure it for downstream processing.
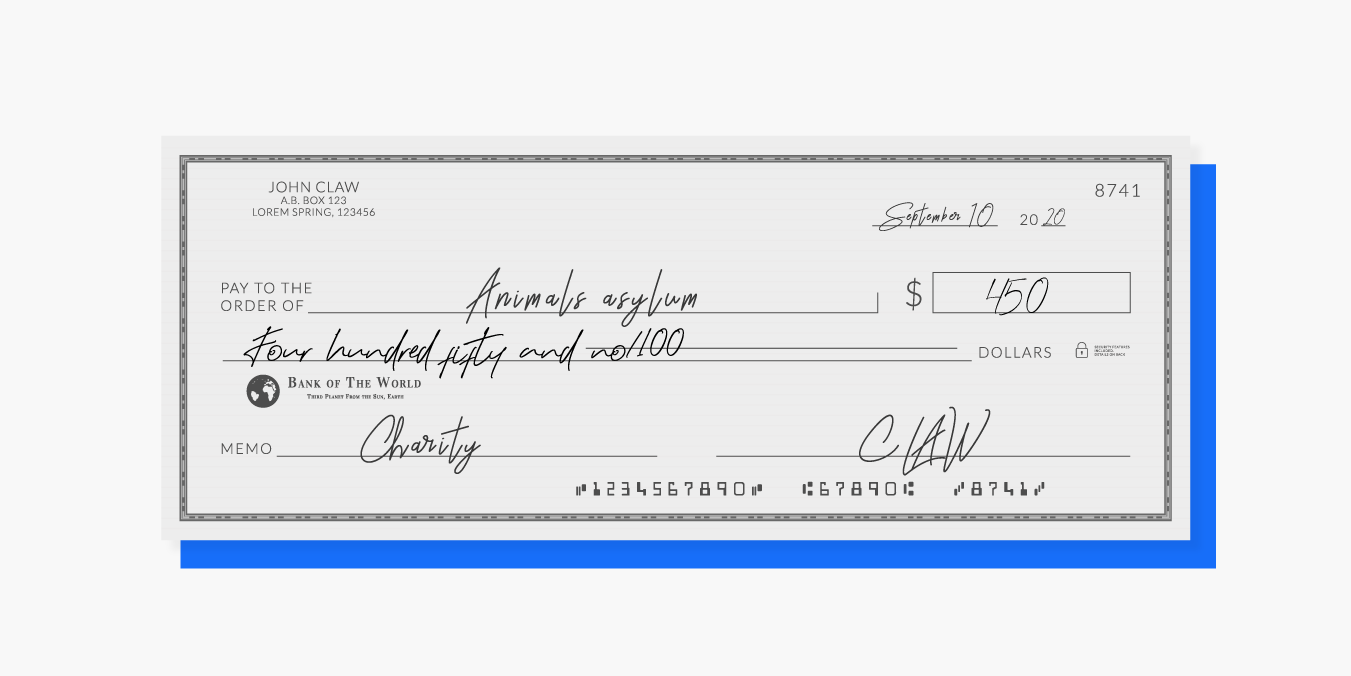
Checks, which require what Hyperscience refers to as a “semi-structured approach” as the data always moves.
- OCR can read the payor address information, check number, and MICR (routing/banking info), but would not be able to capture the handwriting (common for personal checks) for the date, LAR (written out amount in words), and CAR (written amount in numbers).
- Hyperscience uses specialized models to maximize extraction automation and accuracy for checks, which is important for our customers across industries. By reading documents with context (the same way a human would), our solution automatically knows that “four hundred and fifty dollars” is the same as $450.00. If you want to reconcile the LAR and CAR, you’ll need an IDP offering like Hyperscience to read cursive and handprint.