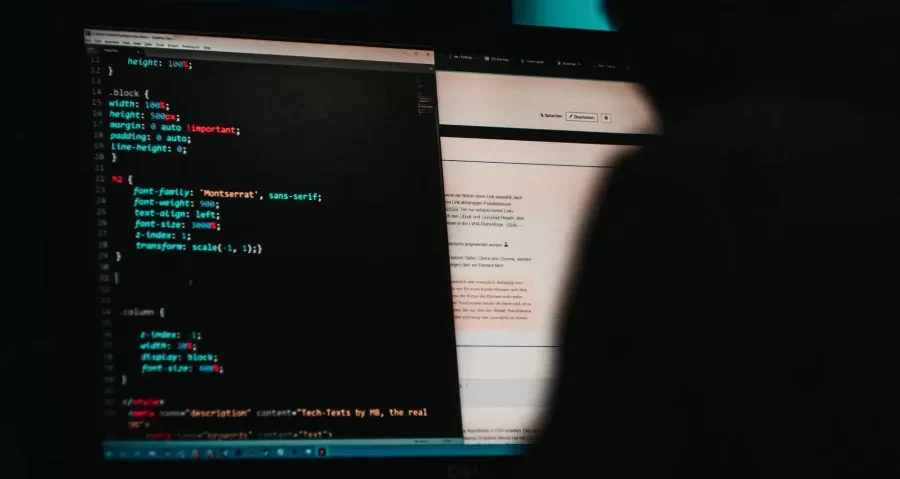
“Data is the new oil.”
This quote from British mathematician Clive Humby is often used to highlight the increasing value and importance of data in today’s digital age. Just as oil was a valuable resource in the industrial era, data has become a valuable asset in the information age. It drives innovation, fuels decision-making, and creates economic value.
But with the increasing importance of data comes the need for responsible handling and protection of personally identifiable information (PII).
To balance the demands of data-driven applications with privacy concerns, synthetic data, the artificial counterpart to real data, has emerged as a powerful solution. In this article, we explore the significance and usefulness of synthetic data in harnessing the power of privacy-preserving insights.
Understanding Synthetic Data
Synthetic data can be defined as artificially generated datasets that mimic real-world data while preserving privacy and confidentiality. It is generally created using tools such as generative models, differential privacy, or data anonymization.
Unlike real data, synthetic data contains no personally identifiable information, reducing the risk of privacy breaches and unauthorized access. As a result, it provides a valuable alternative that enables analysis and innovation without compromising privacy. Because synthetic data addresses privacy concerns so well, Gartner estimates that through 2030, for data used to train AI models, synthetic structured data will grow at least 3x as fast as real structured data.1
The Importance of Synthetic Data
Synthetic data plays a pivotal role in our data-driven world by addressing key data-related challenges. It ensures privacy and data protection, enables data-driven innovation, and helps overcome limitations and biases in real data, promoting fairness and improving decision-making.
Ensuring Privacy and Data Protection
Real data often contains sensitive personal information that can pose privacy risks if mishandled. Synthetic data mitigates these risks by removing all PII, ensuring the privacy and confidentiality of individuals. Researchers, businesses, and organizations can leverage synthetic data for analysis, modeling, and experimentation without infringing on privacy rights.
Enabling Data-driven Innovation
By generating realistic datasets that preserve the statistical characteristics of the original data, synthetic data allows for experimentation and testing of novel ideas and algorithms. Industries such as healthcare, finance, and transportation can leverage synthetic data to develop and refine data-driven solutions while adhering to strict privacy regulations.
Overcoming “Real Data” Limitations and Biases
Due to factors such as sampling biases, underrepresentation, or skewed distributions, real data is susceptible to biases and limitations. Synthetic data helps address these issues by introducing diversity, reducing bias, and enhancing fairness. It enables the creation of more comprehensive and representative datasets, leading to improved decision-making and equitable outcomes.
Challenges and Considerations in Using Synthetic Data
While synthetic data offers significant advantages, there are challenges and considerations to be mindful of as well.
Data Quality and Representativeness
Generating synthetic data that accurately represents the complexity and diversity of the real-world can be a challenge. Techniques such as careful selection of generative models, fine-tuning of parameters, and validation against real data are essential to ensure high data quality and representativeness.
Ethical Considerations and Risks
Ethical considerations arise when generating synthetic data, particularly regarding the source data and the potential for unintended consequences. It is crucial to obtain consent and ensure that the generation process does not perpetuate biases or inadvertently reveal sensitive information. Additionally, boundaries should be put in place to prevent the misuse or misinterpretation of synthetic data, as it may lead to erroneous conclusions or unintended consequences.
Scalability and Computational Requirements
Generating large-scale synthetic datasets that accurately represent the complexity and diversity of real-world data can be computationally intensive and time-consuming. As the size and complexity of the data increase, the computational resources needed for generating, processing, and analyzing synthetic data also grow substantially.
Use Cases and Applications of Synthetic Data
The diverse use cases and applications of synthetic data illustrate its potential to drive innovation and solve real-world challenges in various industries. Here are a few ways that synthetic data can be used within an organization.
Machine learning and AI Model Training
Synthetic data acts as a valuable supplement to real data for training machine learning and AI models. By augmenting existing datasets, synthetic data enhances model performance, generalization, and robustness. It enables better training of models in scenarios with limited or imbalanced real data, leading to more accurate predictions and actionable insights.
Testing and Validation Scenarios
Synthetic data facilitates the creation of diverse and realistic testing scenarios. It allows developers and testers to simulate a wide range of conditions, identify potential edge cases, and ensure the robustness and reliability of applications and systems. Industries like autonomous vehicles, cybersecurity, and healthcare rely on synthetic data to evaluate the performance and safety of their technologies.
Data Sharing and Collaboration
Synthetic data opens up new possibilities for secure data sharing and collaboration. In scenarios where sharing real data is restricted due to privacy concerns or legal constraints, synthetic data provides a privacy-preserving alternative. Researchers, organizations, and stakeholders can collaborate, exchange insights, and innovate without compromising the confidentiality of sensitive information.
By leveraging synthetic data, organizations can navigate the complexities of privacy protection, overcome limitations in real data, and propel industries forward.
Embracing the Opportunity of Synthetic Data
Synthetic data has emerged as a powerful tool in the era of privacy concerns and increasing data-driven demands. By striking a balance between data utility and privacy protection, synthetic data enables innovation, fosters collaboration, and ensures responsible data usage. Its applications span across industries, from machine learning model training to testing and validation scenarios. However, it is crucial to navigate challenges related to data quality, representativeness, and ethical considerations.
To learn more about how Hyperscience can assist with synthetic data generation and its applications, we encourage you to reach out to our team. We believe that through knowledge sharing and collaborating, we can collectively navigate the challenges and opportunities of synthetic data. Let’s explore how synthetic data can enhance your data-driven endeavors while prioritizing privacy and responsible data practices.
- Gartner, Emerging Tech: Venture Capital Growth Insights for Synthetic Data, December 7, 2022
GARTNER is a registered trademark and service mark of Gartner, Inc. and/or its affiliates in the U.S. and internationally and is used herein with permission. All rights reserved.