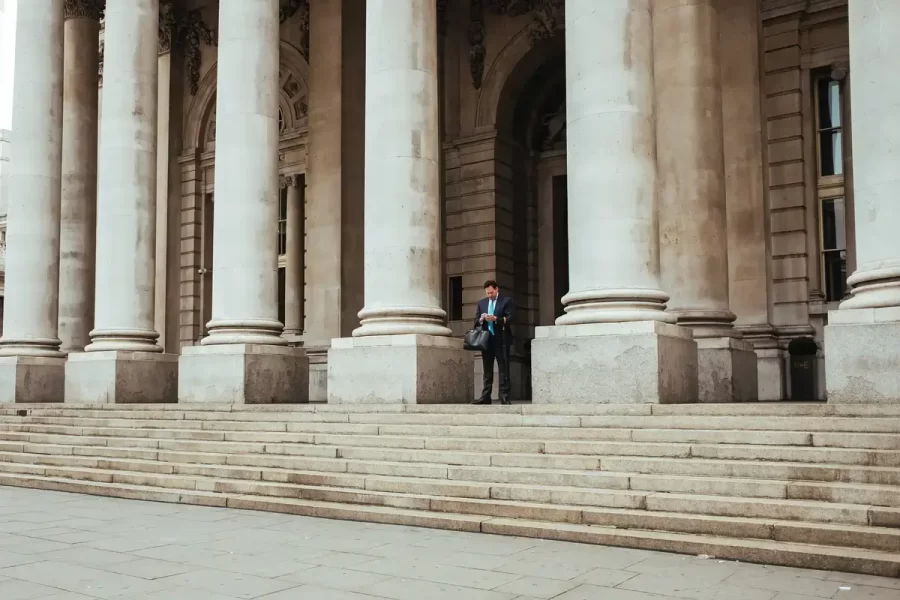
Chris Harr, Federal Sales Director at Hyperscience, recently had the opportunity to join the Federal Tech Podcast to discuss innovation that can help reduce costs for government agencies. Along with host John Gilroy, Chris discusses how intelligent document processing can help federal agencies handle enormous numbers of documents, scaling easily to meet demand.
Episode Transcript:
Narrator: Welcome to the Federal Tech Podcast where industry leaders share insights on innovation for the focus on reducing cost and improving security for federal technology. If you liked the federal tech podcast, please support us by giving us a rating and review on Apple Podcast.
John Gilroy: Welcome to the Federal Tech Podcast. My name is John Gilroy and I’ll be your moderator. Today we have Chris Harr, Sales Director, US Public Sector for a company called Hyperscience: H-Y-P-E-R science, hyperscience.com.
I met Chris a few months back, we talked about his technology, and I think it has a very specific application for the federal audience. I thought we’d get him on here and he could give our listeners a better idea of what Hyperscience does, and maybe bring up an old topic. And the old topic is called OCR: optical character recognition.
Now, an old guy like me, I remember this guy named Ray Kurzweil talking about this years and years ago. For a young guy like Chris Harr, he has a whole different idea of OCR. So I thought, “We need to get the old guy and young guy in the studio and talk this out, and save our federal listeners some money.”
Chris, tell us about your background, maybe a little thumbnail sketch of Hyperscience, and we’ll jump into OCR.
Chris Harr: Thanks for inviting me on the podcast. Since you’ve launched it, I’ve been a longtime listener, and I’ve enjoyed a lot of the episodes that you’ve released.
So I’ve been at Hyperscience for a little over 18 months, but the company has been around since 2014. We’re headquartered in New York City. And we’re actually a series E backed startup. We’ve got just about 300 employees, helping us deliver this technology called “intelligent document processing” to the global markets that we serve. I am on the US Federal team serving as a director here. You know, I think we’ve got a great opportunity in front of us to help the government really eliminate the time tax associated with paper based processes.
JG: You know, I assume that listeners know certain names, and there may be some people listening to this who’ll say “Oh, Kurzweil must be second baseman, you know, for the Phillies or something.” Now, maybe you could tell us more about the genius of Ray, and what he came up with and the legacy today—how it impacts the way we do business.
Outgrowing Optical Character Recognition
CH: Yeah, so Ray Kurzweil; kind of considered the godfather of OCR or optical character recognition, right. And that technology has been around for, you know, three or four decades now at this point. And what that technology did very well was this process of digitization, right?
People would take documents, forms, anything that could be evidence for a claim, and they would scan that document, and they’d run it through OCR, right. And that optical character recognition would help extract some key pieces of data so that you could archive the document or make it easier to retrieve.
But as this process of digitization ballooned, people started to realize that there’s a little challenge here—this pesky thing called scale? If you need to process 1000s of documents a day, you need a different tool to solve that problem.
JG: We know when we’re talking about OCR, and I’m taking notes, and my little brain is kind of going—all three brain cells—I’m thinking, “Well, wait a minute, here’s March, April 2023, what’s going on?” Well, there’s something called taxes coming up. And everyone listening probably has a folder somewhere in their desk with their taxes 2022 on it, and they’re trying to scurry around and get ready.
And guess what? It’s a big paper-based folder. That’s why I bring this up at the beginning of the interview. This whole idea of paper is not some old fashioned topic from 1962. This is important.
And I think when you’re looking at any federal agency, they have to consider the ways they can be more efficient. What can reduce costs? I would think that all this paper floating around has to be one way to reduce costs, isn’t it?
Disruptive Technology: Intelligent Document Processing
CH: Absolutely. You know, going back to the Ray Kurzweil OCR topic, when you talk about scale and tax season, you’re not talking about 10s or hundreds of documents a day. We’re talking about millions, so scale matters.
So when you’ve got millions of documents flowing into your enterprise every single day, the concept of one person opening up every single document, and doing a keyword search over that document becomes incredibly difficult, trying to find out what customer this is for, how much does the person owe? How much are they claiming?—that becomes really difficult.
So this concept of intelligent document processing is taking OCR to the next level using natural language processing and machine learning to solve the context contained in all these data documents, especially with tax returns, to help automate and accelerate, whatever business process this journey is, and I gotta tell you, I hope I get my tax return, or the adjudication of my return done more quickly this year than in years past. And from what I’ve seen out there, it looks like that might happen.
US Chamber of Commerce: Paper Processes Cost Americans $40B
JG: I have some friends coming to town from Alaska—we’re gonna go downtown and maybe see some museums and see what’s going on downtown—and I can remember, once I was downtown, and I had an appointment to talk to the people at the US Chamber of Commerce. It’s it’s kind of like a museum. The building is like a museum. When you walk by it’s like, wow. And the inside the foyer is kind of like “Hey, is this the Smithsonian or something? What’s going on?”
And I just want to make a contrast here, because they have something new, they come up with a study and they said, let’s take a look at this paper stuff. Maybe it’s costing you, taxpayer Chris Harr, or you taxpayer, John Gilroy, more than you think. Why don’t you tell us about this study?
CH: So when the chamber released the study, our team got a kick out of it, because first off, they conducted a fantastic report. But what the report highlights is exactly what our team has been focused on. And it’s, at the end of the day, making work more human by eliminating the time tax associated with document and data processing, through human centered automation.
So in their report, they talk about how eliminating paper-based processes could save taxpayers approximately $40 billion annually.
“Using outdated and manual processes cost Americans an estimated $117 billion and government agencies an estimated $38.7 billion every year.”
U.S. Chamber of Commerce Technology Engagement Center
JG: I read that, and I said, “What? What are you talking about? With a ‘B’?”
CH: I know it’s mesmerizing, right? And they’ve done a tremendous job in the study, they’ve got all these example use cases. And as we read through them, we recognized some of the use cases.
And I gotta tell you, it just makes me think about the market for intelligent document processing, machine learning and natural language processing to help tackle this process of eliminating paper based processes. And, in fact, our top four agency clients, they’re processing nearly a combined 800 million pages annually today through Hyperscience.
JG: So wait a minute, if I’m on Jeopardy, and someone asked me that question, there’s no way I’d guess that. Would you? 800 million? I don’t believe that, Chris.
CH: Yeah, you know, when I was interviewing at Hyperscience, some of the anecdotes that were shared with me were impressive—they caught my attention. And, I think we’re at an interesting time, where new technology brought on by natural language processing and machine learning are helping solve these legacy business challenges.
So I think this staggering number of $40 billion annually is pretty accurate. There might actually be more savings out there for the government and the taxpayer, because I’m telling you from our front row seat and our position in the market, we’ve witnessed firsthand how agencies are addressing the topics in that study, as well as how they’re improving the citizen experience—like through the Biden executive order on citizen experience, which calls out paper based processes taking nearly 9 billion hours of agency time annually.
We’re seeing technology like ours being applied to solve these two challenges.
Natural Language Processing is (Back) in the Spotlight
JG: You just used the phrase, “natural language processing.” I’ve been around here and there a couple times, and I remember this. This was a phrase that was popular, like 15-20 years ago, and it seems to come in and out of style. So what do you mean by natural language processing, especially with your technology?
CH: Well, first off, when you and I were talking a couple of months back, I looked into the history of natural language processing. I looked at Google Trends. And I suggest for your listeners to do this: Look at Google Trends for natural language processing. And you’ll see the topic peaked in February of 2004.
And then for 12 years, it essentially went into this dormant status and interest really didn’t come back until about 2017. And guess what, that’s when Hyperscience really started taking off. That’s when government agencies started contracting with Hyperscience to help them solve their challenges.
And so our team anticipated this interest shift with natural language processing, and so what we’ve done, or what this means to us, is we’ve delivered custom models to our clients over the past couple of years. And just recently, we’ve released a new software update that brings all of our clients text-based document processing in a single unified platform.
So our clients and partners can now unlock valuable insights that were previously trapped in these unstructured documents, thanks to advanced text classification, unstructured extraction, and also data labeling technologies. And that’s all powered by this thing called natural language processing.
What Intelligent Document Processing Means for Your Data
JG: I’m gonna draw a parallel and get in real big trouble here. So in the early days of databases, they were relational. And what’s happened is, they’ve kind of changed over the years. And frequently, today’s modern databases are more object oriented.
Maybe this is what’s going on here; In some ways, in the early days, OCR was just textual. But now, we’ve reached a point where you can actually pull out information that is classifiable, so you go from unstructured to structured, and now you can take unstructured information and scan it in like you couldn’t before. Is this a parallel here, am I off base?
CH: So one area of opportunity for this type of technology is around data analytics, and even predictive analytics. So when you talk about databases, that’s where my mind goes.
If you look at my background, I sold data center infrastructure. I sold big data analytics solutions. And here at Hyperscience, the data that we process sits in that data center. And a lot of the time, customers that we’re talking to, especially in the federal/civilian marketplace, they’re looking at, “Okay, how can I take all of this data that’s now going through this idea of digitalization, how can I take all of the data—all that unstructured data—and put it to use? How can I put it into a usable schema?”
I think that’s one of the magic sauces, if you would, from Hyperscience. Because the data trapped in those old records is only usable if you know exactly what file to look for when you’re using old OCR technology.
And what we’ve done is, we’ve now democratized machine learning, using natural language processing, to find that needle in the haystack—to put it into a usable schema so that you can extract all of the key critical data from your records that can be used more broadly. Maybe it’s mining data to develop additional machine learning models that can predict an FHA or VA mortgage, or maybe it’s to feed conditions-based maintenance predictive analytic models for major airline carriers or even the DOD.
From Structured to Unstructured
JG: Yeah, I think at your website, I read this phrase this morning, “unstructured extraction.” That’s what hit me with unstructured data—that’s, that’s the tough part. I mean, you know, if you have a name, address, phone number, street address, that’s one thing. But some things are much more subtle than that.
CH: Yeah, exactly. And our team sees this as a major area of opportunity. And I’ll tell you what, there are some federal agencies that see this as an opportunity as well. You’ve got the agencies that obviously focus on protecting our borders and helping with immigration, for example.
And some of those agencies, they’ve been in front of the “Chamber” study, they’ve been in front of the Biden executive order on citizen experience, where they’ve actually outlined in their strategic planning, and even with recent RFIs, how they’re going to harness the power of machine learning and natural language processing, specifically through intelligent document processing.
And so agencies that are looking to not only reduce their business backlogs, whether it be FOIA or citizenship, or even tax processing, they should look at intelligent document processing. And a lot of them are, because they recognize their unstructured data can be accurately extracted, and in most cases, automated very quickly
What About Supply Chain Security?
JG: So you’ve mentioned the executive order, and you’ve mentioned the US cybersecurity strategy policies coming up. And now, because it’s in the news, or if you’re at an event and someone walks up to you and says, “Well, what does your scanning have to do with cybersecurity strategy?” Maybe you can bring up two or three points here, and maybe even something related to the supply chain. How does it all tie in?
CH: So I appreciate that you called out the supply chain. I think it’s the fifth pillar–this new cybersecurity policy actually discusses secure supply chains, and John, secure supply chains have been under the microscope for years. I think the first time I remember it really taking center center stage was probably about about 10 years ago, when you saw organizations like Carahsoft, DLT, even Image Group and Arrow looking at secure supply chains.
And agencies—they’ve been focused on how to ensure the goods that they’re purchasing do not have malicious devices, or code embedded in those systems. And by the way, part of that process comes with significant documentation.
And AI Ethics?
But now I see something else happening and some additional legislation coming down the road, John, and that is this idea of secure supply chain from a cybersecurity perspective, but also from a human rights perspective.
And I’ll tell you, you know, Hyperscience just announced our brand new AI ethics steering committee, of which I’m the Public Sector representative, and so you know, we’re taking this topic seriously right, how can our machine learning and AI technology help support these types of topics.
And I think the US government is doing a really good job at putting forth this legislation to give some direction to agencies so that they can protect our critical infrastructure and secure our databases. But there’s a really important piece here. And that is, again, they’re largely document driven. And again, natural language processing can help effectively do that supply tracing.
JG: More and more discussions I have with people about artificial intelligence and machine learning,usually go back to “well, where are you getting your information from? And is there a bias there, or are their conclusions going to be fair?” and I think this is a critique that many people have of this ChatGPT—there are some ethical problems here.
And I never thought about that. To tell you the truth, I never connected the dots between the massive amount of information you have to pour into the machine to get machine learning out of it. It’s not all zeroes and ones—maybe it starts off as paper documents, maybe there’s some censored information that was derived in a laboratory on paper and has to be scanned in. And that can bring a bias to artificial intelligence as well. So maybe that’s where the ethical part fits in. Is that right?
CH: Yeah, you know, I love this conversation. So you’re talking about ChatGPT—you go to the website, they’re taking AI ethics seriously, just like we are. And when you think about, “Okay, how are we going to use tools to solve our business processes?” One thing I think your listeners can consider about Hyperscience is that accuracy matters for the business processes that you’re undertaking.
And I think having the human and the machine teamed together to ensure accuracy of the data that’s extracted that ultimately feeds your downstream business processes, matters. And so the example that you used about some document in the lab going into a process that could affect downstream deliverables—the one thing I’m always going back to here is that with human and machine teaming, you need to have a traceable, auditable record of who touched the data.
And we built that into our platform. You can see if the machine extracted the data, or if a human altered the data in any way. And so traceable data is really key as we think about AI ethics in practice, in the field with our clients and partners.
Let’s Talk Data Labeling
JG: There’s a concept that I’m not real clear on—data labeling, what is that? Where does that fit into the discussion here?
CH: Yeah, so there’s been a lot of talk about data labeling these days, and that’s because to feed an algorithm for machine learning purposes, you need good data in. So data labeling is the practice of telling the machine what piece of data exists, and where it is—classifying it. Some simple examples here; itt’s the practice of teaching the machine that “this image is a picture of a ball or a dog.”
With Hyperscience, it’s the practice of saying “this piece of data is the invoice number, this piece of data is the date the client was seen. This piece of data is the claimants’ details on why they’re submitting a claim.”
And so data labeling is a really serious practice if you want to have accurate, robust algorithms to help predict future processes. Within Hyperscience, we’ve now released this idea of guided data labeling, to accelerate the “humans teaching and machines learning” process within Hyperscience.
How Does Hyperscience Work?
JG: Hey, when we began the discussion, we talked about Ray Kurzweil. I don’t know if you know how big those machines were. But back in the day, those were about the size of a Chevy, and these huge machines have gotten smaller and smaller. And so the product that you have, is it a service? Is it part of a data center? I’m just trying to put my eyes on “Oh, that’s a Hyperscience over there,” and I keep thinking of a big OCR scanner, you know?
CH: So we talked a little bit about magic sauce earlier, and another thing that I think is magical about our software is that we’re containerized. And all of the machine learning and natural language processing—all the magic that happens in our software happens within our clients’ enclave.
So there’s no third party data call needed for you to tap into our full capabilities.There are other capabilities out there that might require a third party data call, but we do not, because of that container.
We operate on Kubernetes and Docker. And so our deployments are on prem, we have a SaaS offering, we’re partnered heavily with AWS. We’ve got some deployments to GovCloud today.
So at the end of the day for people listening that are thinking, “Hey, how can I use natural language processing or machine learning to reduce these paper based processes?”, we’ve got a deployment model that works for them, because every model has security at the front and center.
What’s the Five-Year Plan?
JG: I’m just thinking of several agencies that may be interested in the on premises solution. I can’t name any three letter agencies, but it could work.
But no more three letter agency talk. So billions and billions of dollars, and your job is to reduce the cost for taxpayers. I mean, five years from now, do you think we’re going to catch up on this, or is there gonna be an instant—What’s gonna motivate people to take this technology seriously?
CH: Oh, man, this, you know, we just had our company kick-off, and we had a breakout where everybody was asked to predict the future—what’s gonna happen? There were some fun ones about tax returns, right? Because everybody’s looking at their tax documents right now.
But in five years, I think you’re gonna see the federal government actually taking the lead—getting out in front of the commercial sector in terms of adopting machine learning, and intelligent document processing to digitize their processes.
What does that mean? In terms of future reports coming out of organizations like the US Chamber of Commerce, I think it’d be pretty neat for them to do kind of a retroactive look at how much money has been saved.
I mean, just from one of our deployments, once they go enterprise wide, we’re gonna save them over 50,000 hours of time. And that’s not even one of the larger use cases where, you know, we’re processing nearly 800 million pages annually.
So I do think you’re gonna see the paper based processes being eliminated. We’re gonna see people’s tax returns being processed more quickly. We’re gonna see backlogs for whatever use case come almost to a complete zero here.
JG: So I‘m trying to take this interview and wrap it up with a bow. I talked about doing my taxes earlier, and then you mentioned the phrase kind of casually—”time tax.” So wait a minute, this is all about tax. It’s got nothing to do with the IRS, it’s a time tax involved in handling paper documents.
And so maybe that’s the lesson of this—it’s all about taxes, but nothing to do with those taxes that you send off every year. It’s got to do with the amount of time it takes to process—and many different federal agencies, it could be FEMA, it could be my goodness, NIH, anyone out there—they all have a certain amount of extra burden in handling these documents manually.
It’s gotta be, maybe it is the billion number—seems hard to believe, but let’s find out. We’ll come back in five years and we’ll have a big board, like the amount of money saved in the last five years. No, the number of hours saved, that’s it. The time tax saved over last five years.
You’ve been listening to the federal tech podcast with John Gilroy. I’d like to thank my guest Chris Harr, Sales Director, US public sector Hyperscience.
CH: Thank you, John.
Narrator: Thanks for listening to the Federal Tech Podcast. If you liked the Federal Tech Podcast, please support us by giving us a rating and review on Apple podcasts.