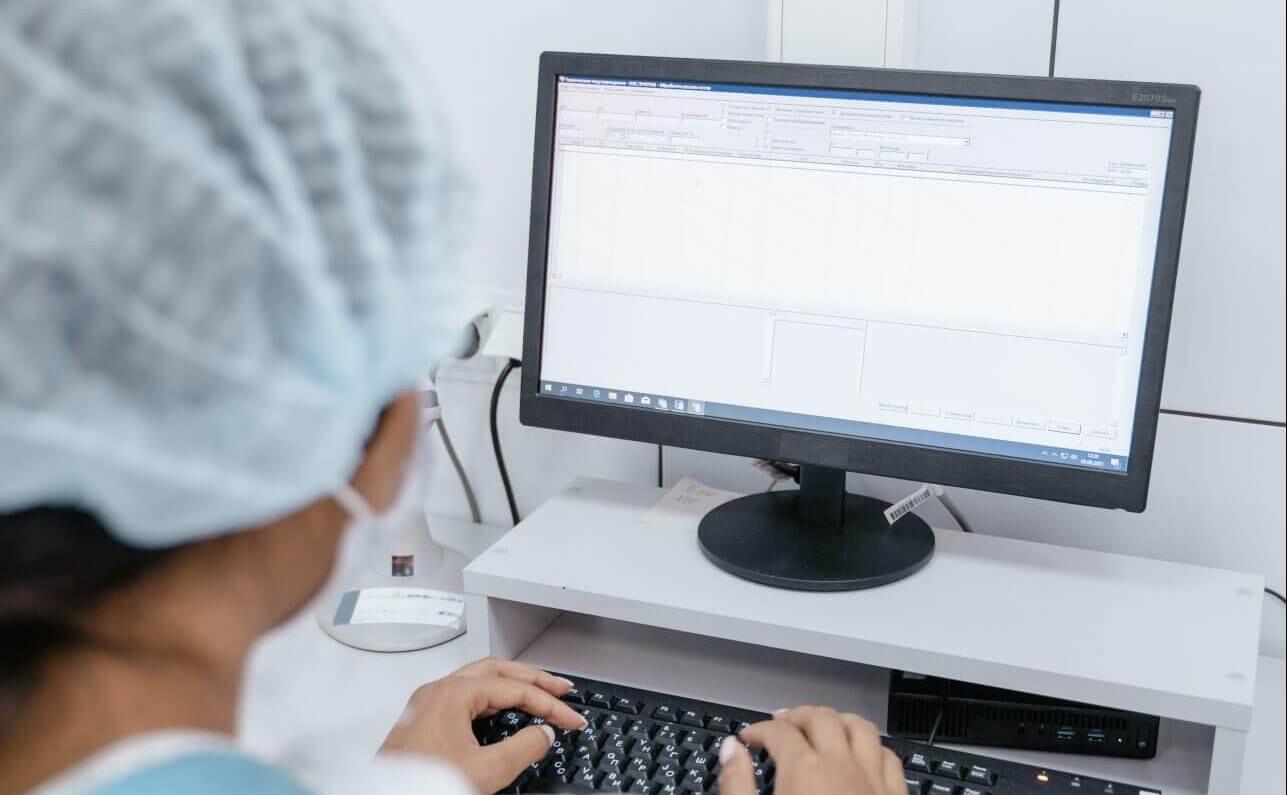
It’s the Difference Between Mutual Enhancement and Simple Collaboration
What is Human-in-the-Loop Machine Learning?
Human-in-the-loop machine learning (HITL ML) is a method of incorporating human input and oversight into the training and decision-making processes of a machine learning model.
This approach can be applied to the automation of complex processes, and involves the integration of human feedback and guidance at various stages of the machine learning pipeline in order to improve the system’s performance. This ensures that it is making decisions that align with human values and objectives.
How is Human-in-the-Loop Machine Learning Different from Attended Automation?
While Human-in-the-loop machine learning and attended automation both aim to improve efficiency through human and machine collaboration, they have some key differences.
With attended automation solutions (such as attended robotic processes automation), all the interactions between human and machine are hardcoded into the logic of the automated process. Some tasks within the process (normally the tedious repetitive ones) are handled by the machine, and others (the complex decisions and exception-handling) are handled by the human.
Imagine an automated invoicing process where a software bot handles the operational tasks. For every invoice over $10,000, this bot has been programmed to include a human manager to manually approve or decline the transaction. The human interacts with the bot, but they don’t learn from each other, they just hand the process back and forth. The process continues running in the same way, day after day, until something changes (like the invoice format changing), and the automation fails.
This human-machine collaboration can definitely save a bunch of hours, but for the complexity of today’s business operations, it’s both too rigid and too fragile. While it combines humans and machines, it fails to enhance the abilities of both.
On the other hand, the use of machine learning and human-in-the-loop to automate complex processes provides a more collaborative and flexible approach—the work done by machines complements and enhances the abilities of humans, and vice versa.
Benefits of Automation Solutions that Combine Machine Learning with Human-in-the-Loop

With the primary difference between the two types of automation out of the way, here are some of the benefits to expect when using HITL ML.
Improved Data Accuracy
With HITL ML, accuracy is the top priority. ML models can recognize their own uncertainty and seek out human input when needed. When the system asks for human input, it uses this feedback to finetune the underlying models, requiring less and less human intervention over time. This improves the adaptability of an organization, leading to more efficient and effective decision making.
Greater Transparency
Machine learning models can be difficult to understand and interpret, especially for non-technical stakeholders. By augmenting the ML model with human input, particularly at the decision-making step, the reasoning behind the model’s decisions can be more easily explained and understood. This can be especially valuable in industries such as healthcare and finance, where trust and transparency are important.
Better Flexibility and User Experience
In some cases, fully automated systems can be inflexible and frustrating to use. By incorporating human workers throughout business processes, organizations can ensure the appropriate training of ML models. This helps businesses automate their processes with the agility needed to adapt to ever-changing market conditions.
Human-in-the-Loop Applications and Use Cases
The following use cases demonstrate how HITL ML can be more effective than attended automation in situations where accuracy, adaptability, and personalized service are important.
Fraud Detection
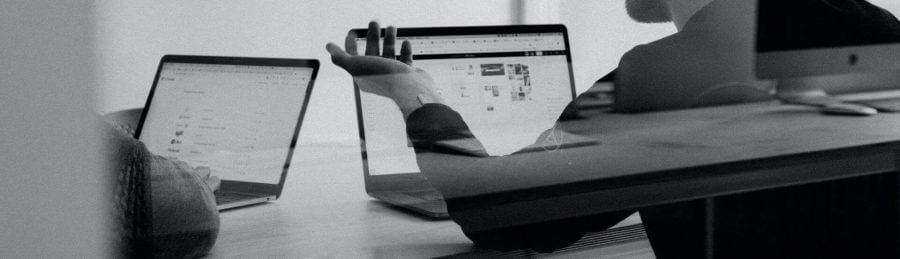
An ML system can analyze large amounts of data and flag suspicious transactions for human review. This approach is more effective than attended automation, which may miss subtle patterns or anomalies that a human would be able to detect.
Customer Service
An ML system can analyze customer data and provide relevant recommendations to a human customer service representative, who can then provide personalized and accurate service to the customer.
Financial Analysis

An ML system can By analyzing market data and provide recommendations to a human financial analyst, who can then make informed decisions about investments or other financial matters.
Benefit Determination
Human-in-the-loop ML can be used to assist with determining eligibility for benefits or services. The system can analyze data from benefit applications and flag potential issues or discrepancies for human review.
Risk assessment
In the insurance industry, HITL ML can be used to assist with risk assessment. An ML system can analyze data from customer profiles and their claim history to provide recommendations to a human underwriter, who can then make informed decisions about risk and coverage.
What’s Next for Machine Learning and Human-in-the-Loop?
When it comes to business productivity and efficiency, HITL ML has the ability to improve an organization’s accuracy, adaptability, transparency, and user experience. And because it’s likely to play a key role in shaping the future of business in the digital age, ML technology should be designed and deployed in a way that lives up to its potential to improve accuracy and efficiency, enhanced by human supervision to ensure accountability and fairness in its outcomes.